Author: Michael "Mike" Turner, CTO, Syntegrity.ai
Introduction
Operational inefficiencies can cost companies valuable time, resources, and money. In mid-cap companies, where resources are often stretched thin, inefficiencies in processes can severely impact overall profitability and competitiveness. Traditionally, businesses have relied on manual methods to identify and resolve operational bottlenecks, but these approaches can be slow and prone to error.
Identifying operational inefficiencies with AI offers a new, more powerful way to identify and resolve these inefficiencies. By automating the process of monitoring, analyzing, and optimizing operations, AI-driven solutions can quickly and accurately pinpoint areas of waste, reduce delays, and streamline workflows. This whitepaper will explore how AI can be used to identify operational bottlenecks and implement solutions that drive efficiency and profitability.
Understanding Operational Bottlenecks
Operational bottlenecks occur when processes within a business are hindered or slowed down by inefficiencies, misaligned workflows, or resource limitations. These bottlenecks can manifest in various forms:
Production Delays: Slowdowns in manufacturing or production due to equipment breakdowns, resource shortages, or inefficient scheduling.
Supply Chain Inefficiencies: Logistical issues that cause delays in procurement, inventory management, or product delivery.
Labor and Resource Management: Bottlenecks in staffing, training, or poor allocation of resources that reduce overall productivity.
Customer Service Gaps: Delays or errors in responding to customer inquiries, which can lead to reduced customer satisfaction and lost revenue.
These bottlenecks can have a cascading effect across the organization, resulting in increased operational costs, reduced output, and lower profitability.
The Role of AI in Identifying Bottlenecks
AI excels at processing large volumes of data and identifying patterns that are difficult for humans to detect. Through continuous monitoring, machine learning algorithms, and predictive analytics, AI can pinpoint bottlenecks before they become critical issues.
1. Real-Time Monitoring of Operations
AI can be deployed to continuously monitor operations in real time, detecting inefficiencies as they occur. For example, in manufacturing environments, AI can monitor equipment performance and identify early signs of malfunction or underperformance. Similarly, in service-oriented businesses, AI can track customer service response times and highlight areas where delays are impacting customer satisfaction.
Example: AI-powered tools used in a factory setting can monitor equipment performance data and automatically trigger alerts when production speeds drop below a certain threshold, signaling a potential bottleneck before it disrupts the production line.
Impact: By using AI for real-time monitoring, businesses can catch inefficiencies early and avoid prolonged delays that lead to significant losses.
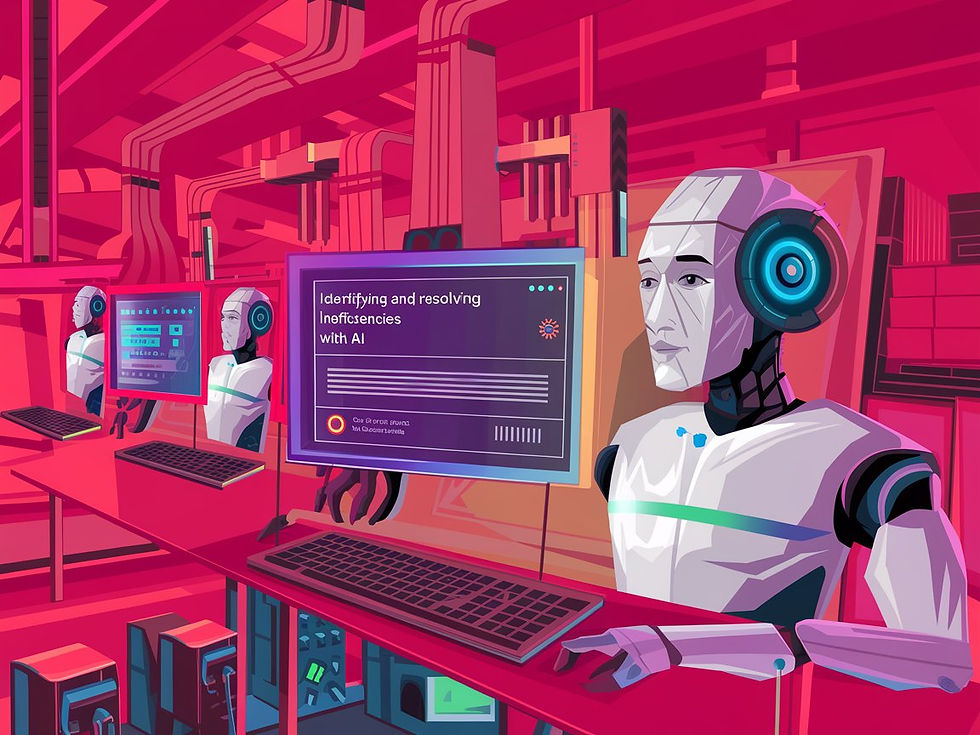
2. Process Analysis and Workflow Optimization
AI can map out entire business processes to identify steps where time, resources, or money are being wasted. Machine learning algorithms can assess workflow data and highlight areas where bottlenecks occur most frequently, whether it’s in production, logistics, or resource allocation.
Example: In a supply chain operation, AI can analyze shipping data to determine the exact point at which delays tend to occur, such as during product distribution or at specific ports of entry.
Impact: With insights into where and why bottlenecks happen, businesses can redesign workflows to eliminate unnecessary steps, reduce delays, and improve overall efficiency.
3. Predictive Analytics for Maintenance and Resource Allocation
AI’s predictive capabilities are instrumental in identifying future bottlenecks before they occur. Predictive analytics allows AI to forecast when equipment is likely to break down, when demand will spike, or when resources will be stretched too thin. This allows businesses to allocate resources more efficiently and schedule preventive maintenance, thereby avoiding downtime.
Example: An AI-driven predictive maintenance system in a manufacturing plant can forecast when specific machinery is likely to experience failure based on historical performance data. The system can schedule maintenance during non-peak hours to prevent disruptions.
Impact: Predictive analytics help companies avoid downtime by anticipating potential problems before they lead to bottlenecks.
The Role of AI in Resolving Bottlenecks
Once operational bottlenecks have been identified, AI can play a crucial role in resolving them by automating workflows, optimizing resource allocation, and enhancing decision-making.
1. Workflow Automation
AI can automate repetitive or time-consuming tasks, freeing up human resources to focus on higher-value activities. By automating key parts of the workflow, businesses can reduce the risk of bottlenecks caused by human error or resource constraints.
Example: In customer service, AI-powered chatbots can handle routine inquiries, allowing human agents to focus on more complex issues. This reduces wait times and prevents bottlenecks in customer support channels.
Impact: Automating routine tasks reduces inefficiencies and accelerates the flow of work, resulting in fewer delays.
2. Dynamic Resource Allocation
AI can optimize resource allocation by analyzing data on demand, resource availability, and productivity. AI-driven systems can dynamically adjust staffing levels, equipment usage, or material allocation to ensure that resources are always used efficiently and bottlenecks are minimized.
Example: AI can be used in a retail environment to optimize staffing levels based on real-time customer traffic data, ensuring that there are always enough employees during peak hours to prevent slowdowns in service.
Impact: Dynamic resource allocation enables businesses to be more agile, reducing the risk of bottlenecks caused by under- or overutilization of resources.
3. AI-Driven Decision Support Systems
AI-powered decision support systems (DSS) can provide managers with real-time insights and recommendations for resolving operational bottlenecks. These systems analyze operational data and offer solutions based on historical performance, predictive models, and current business conditions.
Example: In logistics, an AI-driven decision support system might recommend alternative shipping routes during times of high demand to avoid delays caused by traffic congestion or weather disruptions.
Impact: By providing real-time insights and recommendations, AI-driven DSS can empower managers to make more informed decisions and resolve bottlenecks before they become critical.
Real-World Use Cases: AI in Identifying and Resolving Operational Bottlenecks
Use Case 1: AI in Manufacturing
Company: Siemens AI Application: Predictive maintenance and process automation.
Outcome: Siemens uses AI-driven systems to monitor the performance of its machinery in real-time. By identifying inefficiencies and predicting equipment failure before it occurs, Siemens has been able to reduce downtime by 20% and improve overall production efficiency.
Impact: The integration of AI into their operations allowed Siemens to identify bottlenecks early, streamline production workflows, and improve the efficiency of their manufacturing processes.
Use Case 2: AI in Supply Chain Management
Company: DHL AI Application: Supply chain optimization using predictive analytics and dynamic routing.
Outcome: DHL implemented AI to predict potential delays in their supply chain and automatically reroute shipments based on real-time data. This allowed DHL to improve delivery times and reduce the occurrence of bottlenecks in their logistics operations.
Impact: By using AI to anticipate and resolve bottlenecks, DHL was able to streamline its supply chain operations, leading to higher customer satisfaction and reduced costs.
Steps for Mid-Cap Companies to Implement AI for Bottleneck Resolution
1. Conduct a Bottleneck Assessment
Before implementing AI, companies need to conduct an assessment of their current operational bottlenecks. Identify which processes or departments are experiencing inefficiencies and where delays are most likely to occur.
2. Invest in AI Tools for Monitoring and Analytics
Mid-cap companies should invest in AI tools that offer real-time monitoring and analytics capabilities. These tools should be capable of tracking key performance metrics, identifying bottlenecks, and providing actionable insights.
3. Train Teams to Utilize AI-Driven Insights
To maximize the value of AI, it’s essential that teams are trained to interpret and act on AI-driven insights. This includes empowering managers with the skills to use decision support systems and predictive analytics to optimize operations.
Conclusion: Resolving Operational Bottlenecks with AI
AI provides mid-cap companies with the ability to identify and resolve operational bottlenecks more effectively than traditional methods. By leveraging real-time monitoring, predictive analytics, and automation, AI can streamline workflows, optimize resource allocation, and enhance decision-making processes. Companies that integrate AI into their operations can expect to see significant improvements in efficiency, productivity, and profitability.
Operational bottlenecks no longer need to be a hindrance. With AI, businesses can anticipate and resolve inefficiencies before they impact overall performance.
Yorumlar