Author: John Martin, CFO, Syntegrity.ai
Introduction
In today’s hyperconnected world, businesses face a complex and evolving risk landscape. From cybersecurity threats to market volatility, unforeseen risks can derail even the most successful companies. Traditionally, businesses have relied on human-led risk management strategies, which often involve manually identifying potential threats, assessing their impact, and mitigating them. However, as risks become more dynamic and difficult to predict, Artificial Intelligence (AI) is emerging as a powerful tool to enhance risk management practices.
AI-driven risk management enables companies to predict, detect, and mitigate risks in real-time, making it possible to respond proactively to unforeseen threats. This whitepaper explores how AI is transforming risk management, providing companies with advanced tools to protect their assets, financial health, and operations from unexpected dangers.
The Evolving Risk Landscape
Modern businesses face a wide variety of risks, including:
Cybersecurity Threats: As companies increasingly move operations online, they are exposed to cyber-attacks, data breaches, and ransomware.
Financial Risks: Market volatility, credit risk, and liquidity concerns continue to threaten financial stability, particularly for mid-cap companies with limited risk buffers.
Operational Risks: Supply chain disruptions, equipment failures, and regulatory non-compliance can create operational bottlenecks that negatively impact a company's performance.
Reputation and Compliance Risks: Missteps in data management, regulatory compliance, or public relations can damage a company's reputation and lead to legal consequences.
Traditional risk management frameworks struggle to keep up with the complexity and speed of modern risks. Enter AI, a solution that enhances risk detection, forecasting, and mitigation by processing vast amounts of data in real-time, allowing businesses to anticipate threats before they materialize.
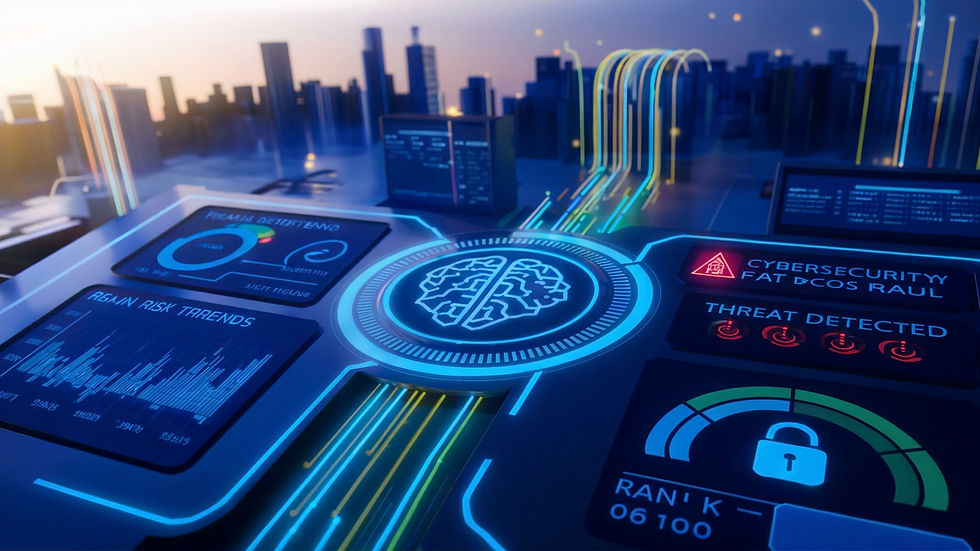
How AI Transforms Risk Management
1. Predictive Risk Identification
AI’s predictive capabilities allow businesses to detect potential risks before they escalate into full-blown crises. By analyzing historical data, market trends, and external events, AI can forecast future risks and alert decision-makers to areas of concern.
Financial Risk Prediction: AI-driven financial models can analyze macroeconomic indicators, company financials, and market data to predict potential downturns or liquidity issues. For example, AI can forecast the likelihood of a credit default by analyzing a borrower’s financial health and past credit behavior.
Operational Risk Detection: AI can identify operational risks by monitoring real-time data on supply chains, production processes, and equipment performance. Machine learning algorithms analyze this data to flag anomalies that indicate potential breakdowns or inefficiencies.
Impact on Risk Management: Predictive analytics helps businesses move from a reactive risk management model to a proactive one, allowing them to take preemptive action to avoid or mitigate risks.
2. Real-Time Threat Detection and Response
One of the greatest strengths of AI is its ability to analyze and respond to data in real-time. This capability is crucial in environments where risks—such as cybersecurity threats—evolve rapidly and require immediate attention.
Cybersecurity: AI-driven tools can detect unusual activity in IT systems by analyzing vast amounts of data for signs of anomalies, malware, or unauthorized access. AI-powered systems continuously monitor network traffic and user behavior to identify threats like phishing attacks, ransomware, or insider threats in real-time. When an attack is detected, AI can automatically isolate compromised systems and notify security teams for rapid resolution.
Supply Chain Monitoring: AI can monitor supply chains to detect disruptions, such as delays caused by extreme weather, geopolitical events, or supplier insolvencies. By analyzing external factors in real time, AI can recommend alternative suppliers or transportation routes, ensuring continuity in operations.
Impact on Risk Management: Real-time threat detection enables businesses to respond instantly to potential risks, reducing the impact of cyber-attacks, supply chain disruptions, and operational breakdowns.
3. Automating Compliance and Regulatory Risk Management
Compliance with ever-changing regulations is a significant challenge for businesses, particularly those in highly regulated industries such as finance, healthcare, and manufacturing. AI can streamline compliance processes by:
Automating Data Audits: AI systems can automatically audit financial transactions, healthcare records, or operational logs to ensure compliance with industry regulations.
Monitoring for Regulatory Changes: AI-powered systems can continuously scan legal and regulatory databases to identify changes that may impact a company’s operations. When new regulations are introduced, AI can automatically assess their relevance and recommend actions to ensure compliance.
Impact on Risk Management: Automating compliance tasks reduces the risk of regulatory violations, helping businesses avoid fines, legal battles, and reputational damage.
Real-World Use Cases: AI in Risk Management
Use Case 1: AI in Financial Risk Management
Company: HSBCAI Application: AI-driven financial risk modeling and fraud detection.
Outcome: HSBC uses AI to detect financial fraud by analyzing millions of transactions in real-time, identifying patterns that suggest fraudulent activity. By leveraging machine learning algorithms, HSBC can prevent financial fraud and enhance credit risk analysis, enabling more accurate predictions of default risks.
Impact on Risk Management: HSBC has reduced fraud losses and improved its ability to forecast credit risks, enabling it to manage financial threats more effectively.
Use Case 2: AI in Cybersecurity
Company: DarktraceAI Application: AI-powered cybersecurity threat detection and response.
Outcome: Darktrace uses AI to monitor corporate networks in real-time, detecting potential cyber threats before they escalate. The AI-driven system autonomously responds to attacks, isolating compromised systems and neutralizing the threat while minimizing disruption to the business.
Impact on Risk Management: Darktrace’s AI solution has helped businesses reduce the time it takes to identify and respond to cybersecurity threats, mitigating the risk of large-scale data breaches.
AI-Driven Risk Management Tools: Key Areas of Application
1. AI-Powered Predictive Analytics for Financial Stability
AI can be used to develop advanced predictive models that assess financial stability and forecast risks such as:
Credit Risk: By analyzing credit history, transaction data, and macroeconomic factors, AI can predict the likelihood of defaults or credit downgrades.
Liquidity Risk: AI models can forecast cash flow fluctuations and liquidity risks, helping businesses ensure they have enough cash on hand to meet obligations.
2. AI-Driven Fraud Detection Systems
AI excels at identifying suspicious transactions or behaviors that may signal fraud. AI-driven fraud detection systems continuously monitor transaction data, using machine learning to spot patterns that deviate from normal behavior. These systems can:
Detect Payment Fraud: In industries like retail and banking, AI can detect fraudulent credit card transactions in real-time by identifying anomalies in purchase patterns.
Identify Insider Threats: AI can monitor employee behavior for signs of insider threats, such as unauthorized access to sensitive data.
3. AI for Regulatory Compliance
AI-driven compliance tools automate the monitoring of regulatory requirements and ensure that businesses remain compliant with local and international laws. AI can audit company data to detect areas of non-compliance, flagging potential issues before they lead to penalties.
Steps for Mid-Cap Companies to Implement AI for Risk Management
1. Identify Key Risk Areas
Businesses should first assess their risk landscape to identify which areas—whether financial, operational, or cybersecurity—are most vulnerable to unforeseen threats. This will help prioritize AI implementation.
2. Select AI Tools for Risk Management
Once risk areas are identified, companies should invest in AI tools that address their specific needs. These tools might include AI-driven financial risk models, fraud detection systems, or cybersecurity monitoring solutions.
3. Integrate AI into Existing Risk Management Frameworks
AI should be integrated into the company’s overall risk management framework to complement existing processes. This may involve training teams to interpret AI-driven insights and updating policies to reflect AI’s capabilities.
Conclusion: Protecting Your Business from Unforeseen Threats with AI
AI offers businesses the ability to predict, detect, and mitigate risks in ways that traditional risk management frameworks cannot match. From forecasting financial risks to detecting cybersecurity threats in real time, AI provides the tools necessary to stay ahead of unforeseen challenges. Companies that invest in AI-driven risk management systems will not only protect their assets but also build a more resilient and adaptable business in the face of an increasingly unpredictable risk landscape.
Comments